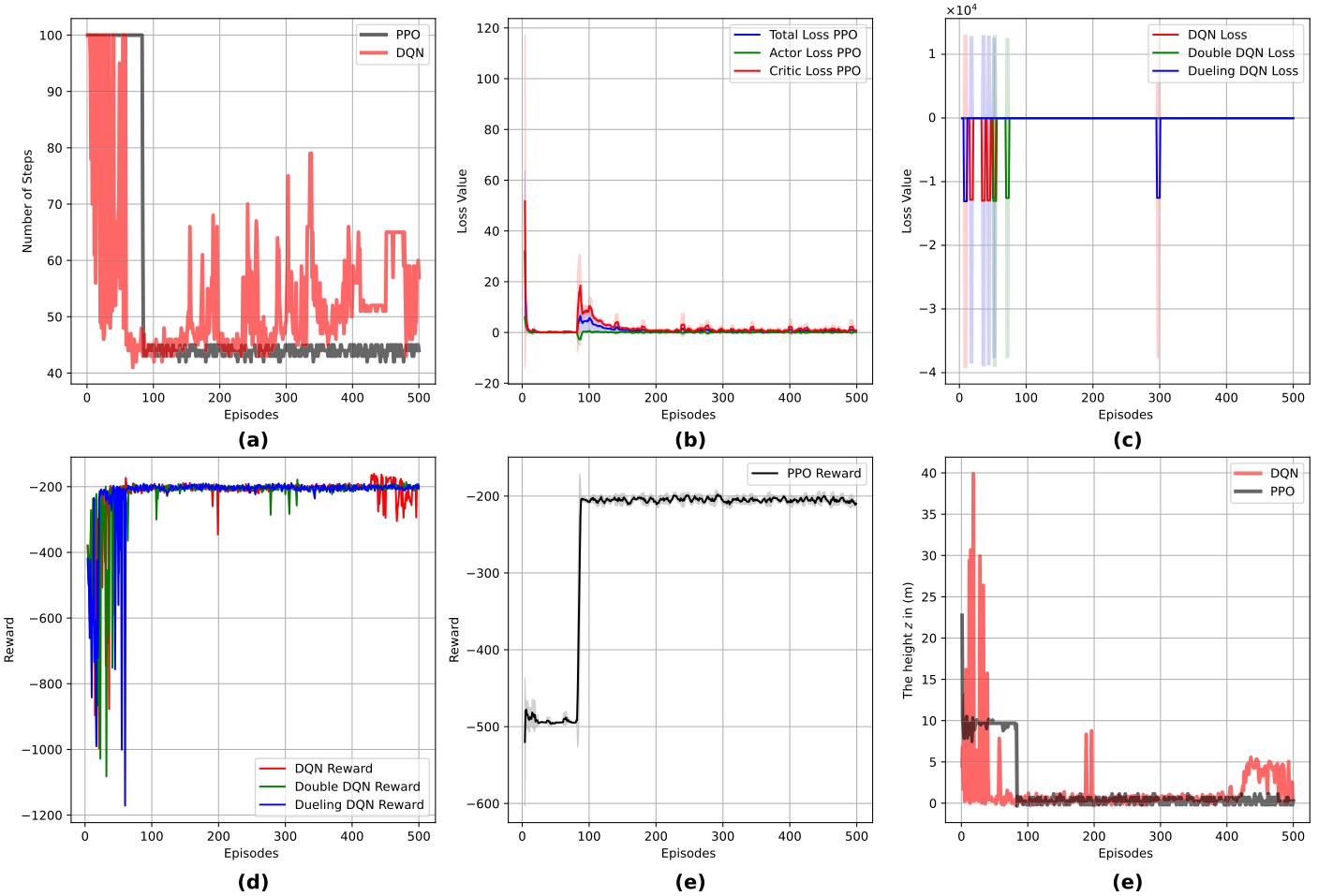
|
Fig. (a) compares the number of time steps needed to land for all the agents. (b) illustrates the actor and critic loss moving average value of the PPO agent, while
(c) shows the moving average loss value of the DQNs agents. (d),(e) depict the moving average of the reward of DQNs and PPO agents respectively. Finally,
(f) compares the final height achieved by DQN and PPO agents. The shaded parts in all figures represent the standard deviation of the moving average.
|
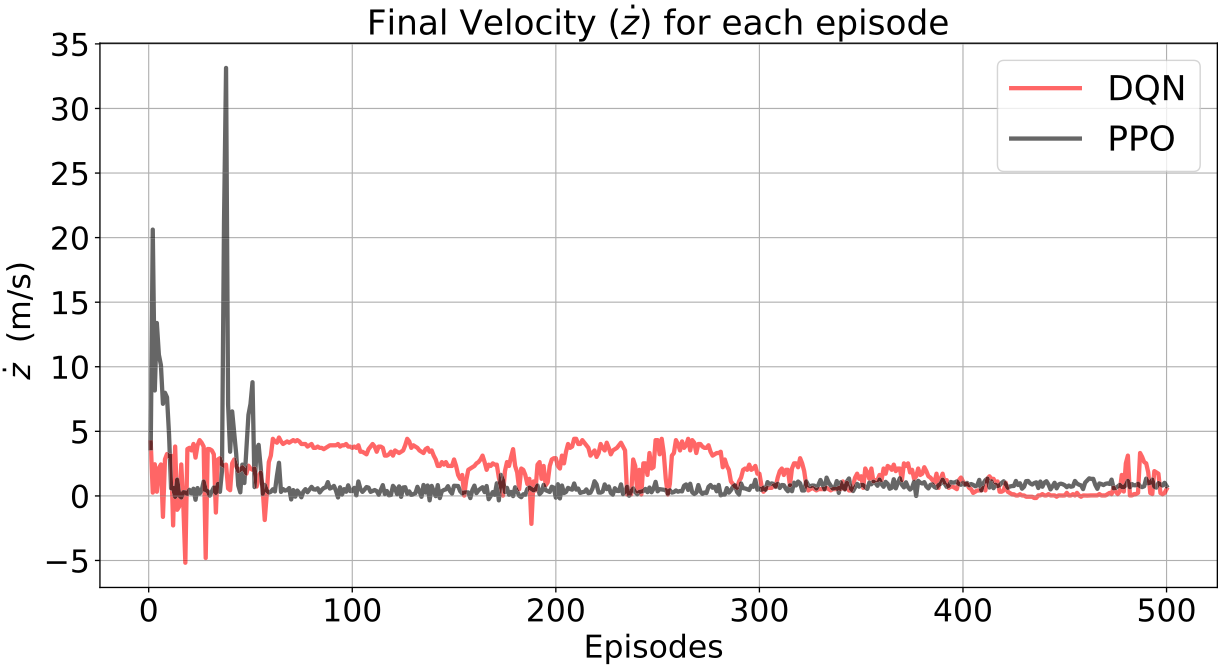
|
Fig. Comparison between the final impact velocity of the VTOL-UAV in the case
of PPO and DQN agents.
|
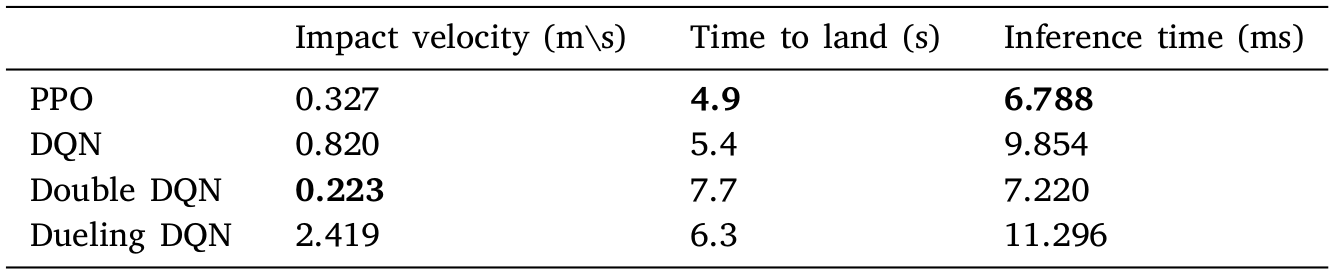
|
Fig. Evaluation of the trained agents.
|